Excellent question! After all, does simply adding a G to AI make that much of a difference? The fact is that the G in Artificial General Intelligence is as unnecessary as the A in Artificial Intelligence. Intelligence is intelligence, its origins are of secondary importance.
However, we can’t ignore the fact that Artificial Intelligence has become, at least in many circles, practically synonymous with ML/DL and statistical learning. Hence, in order to clearly distinguish today’s pattern recognition technology from “thinking machines” that are comparable to the human mind in many non-trivial aspects, the term AGI is helpful. Perhaps some day in the future we will find ourselves more concerned with Intelligence independent of whether it is natural or artificial. This is the focus of some of my blogs based on Korzybski’s General Semantics which is probably one of the most advanced inquiries into the nature of intelligence in general.
Let’s assume for the sake of argument that, at least for the moment, AI = ML/DL = statistical learning. Deep learning is an amazing advancement enabled by the convergence of several factors:
- Low Cost and Ubiquitous High Performance Computing (HPC) (SIMD specifically) — approximately 10 years ago NVIDIA revolutionized this space with GPGPU’s
- Massive amounts of data — aka “the internet” and other developments led to the creation of massive data lakes… largely unused
- Improved algorithms — Hinton, LeCun, Bengio, Schmidhuber, Ng and other luminaries led the charge to create improved algorithms capable of making use of the HPC + data
When the representations that DL learns are analyzed, there is even more reason to be optimistic about the possibility that DL can lead to AGI.
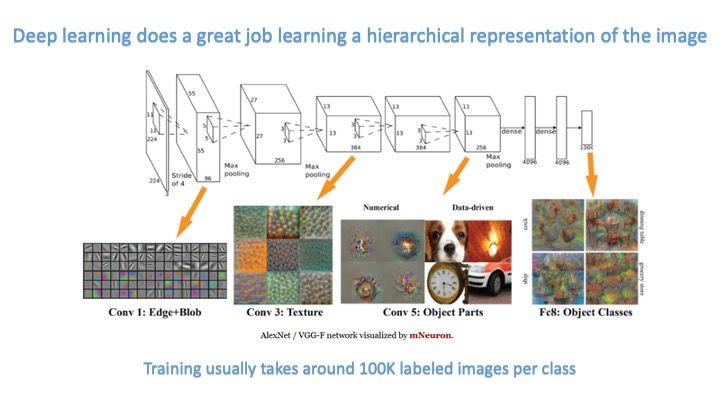
The hierarchical knowledge representations that DL learns have some similarities to those learned by animal and human brains. Fantastic! So what’s the problem?
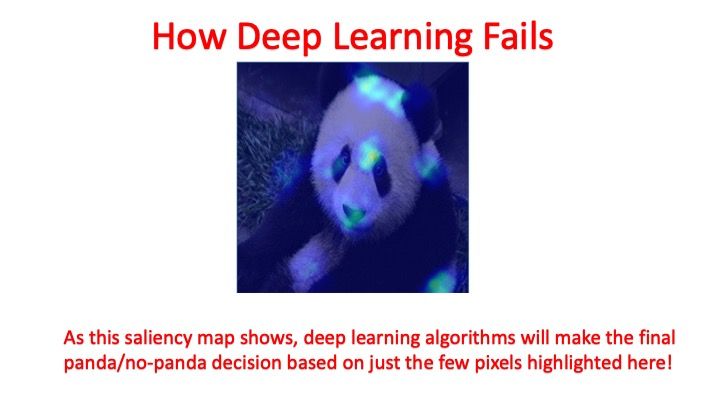
The problem is that current DL algorithms tend to utilize only a few pixels to make the final classification decision. This means that even though DL models include deep hierarchical information, the ultimate performance of the DL classifier is based on a rather shallow knowledge representation. This is why it is easy to fool many DL algorithms with adversarial examples that can reliably turn a correct Stop Sign classification into a Speed Limit X classification. How can we improve this situation?
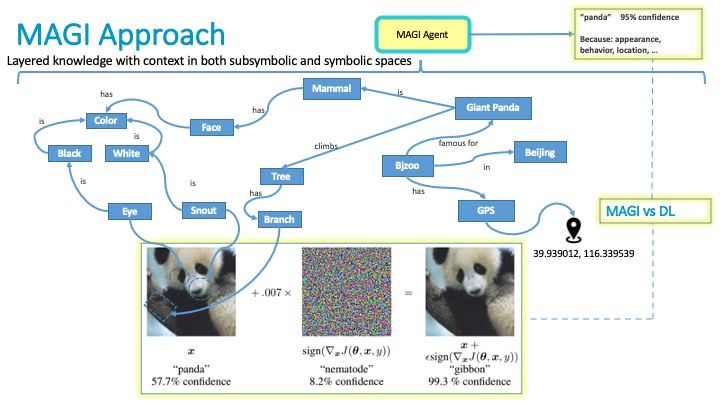
In a nutshell, by combining the deep hierarchical knowledge representation learned by the deep learning system with a symbolic knowledge representation. A quick google search for neurosymbolic or knowledge graph will yield many hits. We’ve learned a few things over the years about these sorts of neurosymbolic systems in practice which you can learn more about here:
https://www.researchgate.net/project/A-Metamodel-and-Framework-For-AGI. In a word, we’ve learned that simply gluing together ML/DL and a knowledge graph is not quite enough. In order to achieve the holy grail “blessing of dimensionality”, you need to add some additional structure.
Our initial paper above is a good starting point for learning more about the nature of this additional structure. My plan is to cover the key points in a series of additional medium blogs. Of particular interest is that this additional structure appears to be beneficial to intelligences in general be they “natural” or “artificial”.