INSIGHTS

8 min read
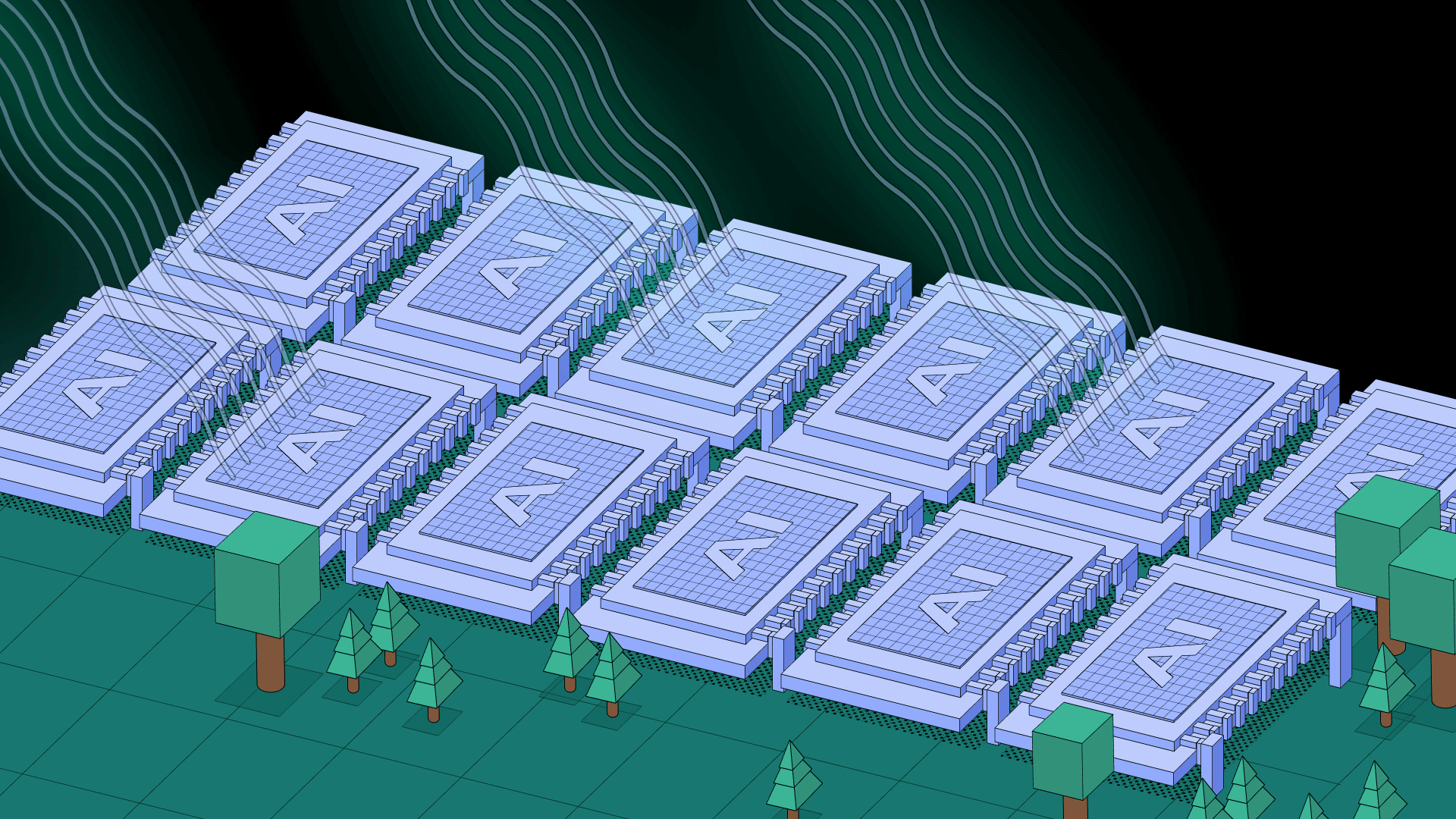
Published on 06/19/2024
Last updated on 06/19/2024
How your organization can integrate sustainability into AI development
Share
One of the most encouraging use cases for artificial intelligence (AI) to date is its potential to address the climate crisis. Trained on vast datasets, AI models can, for instance, guide businesses toward more energy-efficient processes and sustainable materials or advance the development of renewable energy technologies. Governments can also lean on AI to forecast environmental disasters and plan appropriate responses.
Discussions around these capabilities are hopeful and captivating, but there’s less talk about the inherent environmental costs of training, using, and maintaining AI systems themselves. AI models have significant energy demands that go beyond what is required for other types of computing tasks. Right now, data centers where large language models like OpenAI’s GPT-4 are trained and deployed account for between 1% and 2% of global electricity consumption.
As the AI field grows, resource demand will rise. Researchers, developers, legislators, and the public are examining the technology’s implications, a process that will likely continue for years. However, while policymakers around the world are making progress when it comes to AI governance and safety, few of them have developed comprehensive guidelines around AI’s environmental footprint.
Defining sustainable AI and understanding its environmental impacts
When talking about AI and sustainability, it’s important to make the distinction between AI for sustainability and the sustainability of AI. While some AI practitioners use the technology to support sustainability efforts, the sustainability of AI refers to the environmental impacts of AI lifecycles.
An early definition by researcher Aimee van Wynsberghe describes the latter: “Sustainable AI deals not exclusively with the implementation or use of AI but ought to address the entire lifecycle of AI, the sustainability of the: design, training, development, validation, re-tuning, implementation, and use of AI.” This lifecycle uses significant resources, including electricity and water, which will only increase in the years ahead.
Energy consumption
Large AI models require substantial computing power to develop, use, and maintain the technology. Research from 2019 indicates that training a natural language processing (NLP) model can require 600,000 pounds of carbon emissions, which is equivalent to the emissions produced by five cars over their entire lifetime. This resource consumption is also quite condensed over short training periods. For example, Google’s AlphaGo Zero generated 96 tons of carbon dioxide over 40 days of training, comparable to 1000 hours of air travel.
Water
Hydropower is often used to generate electricity for AI model development. Data centers where models are trained and deployed produce a lot of heat and require water to stay cool. Early in the AI lifecycle, items like the chips and servers that make up AI infrastructure also use a lot of water in the semiconductor manufacturing process. Rising global demand for AI is forecast to require between 4.2 to 6.6 billion cubic meters of water withdrawal by 2027—half of the United Kingdom’s annual consumption. Much of the water used to fuel the AI lifecycle is also potable, raising ethical concerns as global water shortage risks rise.
Electronic waste
As AI innovations evolve at an unprecedented pace, hardware will continually become outdated. Disposal of this tech can mean that hazardous materials, including heavy metals like lead and cadmium, leach into the environment, impacting surrounding ecosystems and communities.
Navigating the environmental impact in AI innovation and regulations
To lead change in more responsible AI practices, enterprises must stay compliant with regulations governing the technology and anticipate evolving rules around more sustainable AI lifecycles.
Several laws and guidelines covering AI data governance, security, and safety have been published in different jurisdictions—including the General Data Protection Regulation (GDPR), the European Union’s AI Act, and the White House’s executive order on AI development. However, there are currently no documents with comprehensive protocols on AI development and the environment.
The EU AI Act has some verbiage around sustainability, but only for high-risk models in critical sectors like healthcare, transportation, and law enforcement. As more organizations create an environmental footprint through AI development, enterprise leaders must treat legislative gaps as an urgent strategic challenge.
There have been some moves to create a regulatory framework around AI and environmental protection. For example, the Artificial Intelligence Environmental Impacts Act of 2024 is a new bill that calls for the Environmental Protection Authority (EPA) to investigate impacts across AI lifecycles. It also requests that the National Institute of Standards and Technology (NIST) create measuring and reporting standards for managing AI impacts on the environment.
Researcher Philipp Hacker has proposed the concept of sustainability by design, which would require tech companies to use training and deployment frameworks that are built intentionally to reduce environmental risks. Hacker also suggests making impact assessments mandatory for all models under the EU AI Act—not just those categorized as high-risk.
As the regulatory space evolves, organizations must be prepared to adapt. This could mean complying with energy consumption caps or minimum renewable energy requirements during model training.
Support your commitment to sustainable AI
Every organization will have different values, goals, and resources for environmental protection, so strategies to ensure sustainable AI development will vary widely. While legislative compliance is critical, practices like emissions tracking and innovative training techniques can give enterprises a competitive edge in areas like environmental, social, and governance (ESG) investment and consumer trust.
Emissions tracking and transparency
It’s difficult to set goals around the sustainability of AI without first evaluating your environmental footprint. Some factors to consider are the type of hardware you use, how much energy the model consumes, where your infrastructure is located, and the length of training periods. There are also several emissions tracking tools currently available with varying techniques and levels of accuracy:
Maintaining a record of your AI infrastructure and impact metrics will allow you to assess your model’s environmental footprint over time. This facilitates ESG reporting and offers greater transparency for employees, consumers, and stakeholders.
Model validation
AI models are being trained for a wide range of applications, from gaming to healthcare. As the climate crisis escalates, questions may arise around the validity of some of these use cases, considering the environmental cost of their development.
Once your organization has determined how it wants to leverage AI and what the associated environmental footprint is, the next step is to weigh these factors against your values. Determine whether the model’s outcomes are worth AI’s environmental impact or if there is a more beneficial use case that would make its impact more justifiable.
Efficient training techniques
More efficient model training techniques can help address both the limited availability of computational resources and environmental concerns. For instance, “pause and resume” algorithms can temporarily stop AI workloads when emissions in the local area are high. Low-powered hardware, such as that which supports the TinyML framework, can also reduce power consumption by up to 1000x when compared to regular graphics processing units (GPUs). In addition, innovations like quantum computing could be useful for model training and energy efficiency in the future.
Researchers at the MIT Lincoln Laboratory Supercomputing Center are also exploring ways to make AI computing more efficient. For example, GPUs manufactured to draw less power can lower energy consumption by 12% to 15% and reduce cooling requirements. To support machine learning operations (MLOps), the Center has developed hardware optimization techniques, like switching between high-power GPUs and low-power central processing units (CPUs) based on fluctuating computing needs. The team has also been successful with techniques that can identify and stop underperforming models early on in their training to save energy. Such efficiency gains can help enterprises reduce computing costs and address sustainability goals while innovating with AI.
Responsible providers
Your AI lifecycle likely involves several players, from cloud service providers to hardware manufacturers (if you’re building your own computing infrastructure). Ensure third parties, with their varying environmental practices and values, align strategically with your standards when developing your AI infrastructure. Consider things like how transparent they are about their emissions or if they’re delivering on sustainability commitments with solutions like AI for renewable energy or more efficient technologies.
Become a leader in the sustainability of AI
Because we’re in the early stages of a major AI transformation, standards around environmental stewardship still have a long way to go. This presents a strategic opportunity for enterprises to take the initiative and position themselves at the forefront of responsible AI development.
Establishing clear values, standards, techniques, and reporting strategies for more sustainable AI lifecycles can make a difference not only to the planet. It will also differentiate your organization as an AI practitioner and help you earn trust as the landscape evolves.
Learn more about Outshift’s commitment to trustworthy and responsible AI.
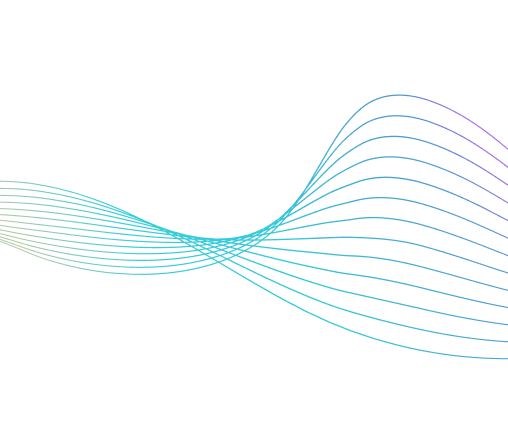
Get emerging insights on emerging technology straight to your inbox.
Unlocking Multi-Cloud Security: Panoptica's Graph-Based Approach
Discover why security teams rely on Panoptica's graph-based technology to navigate and prioritize risks across multi-cloud landscapes, enhancing accuracy and resilience in safeguarding diverse ecosystems.
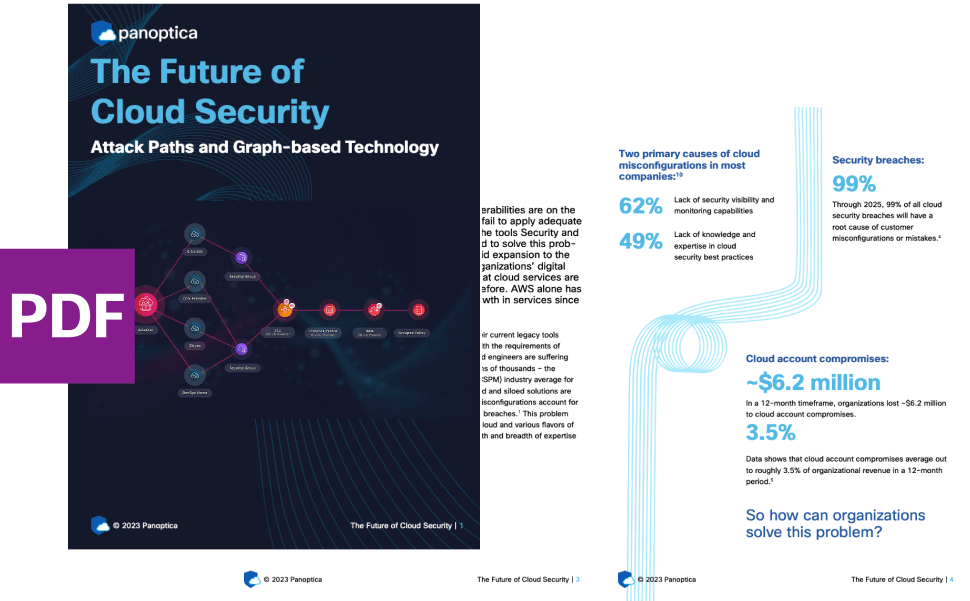
Related articles
The Shift keeps you at the forefront of cloud native modern applications, application security, generative AI, quantum computing, and other groundbreaking innovations that are shaping the future of technology.
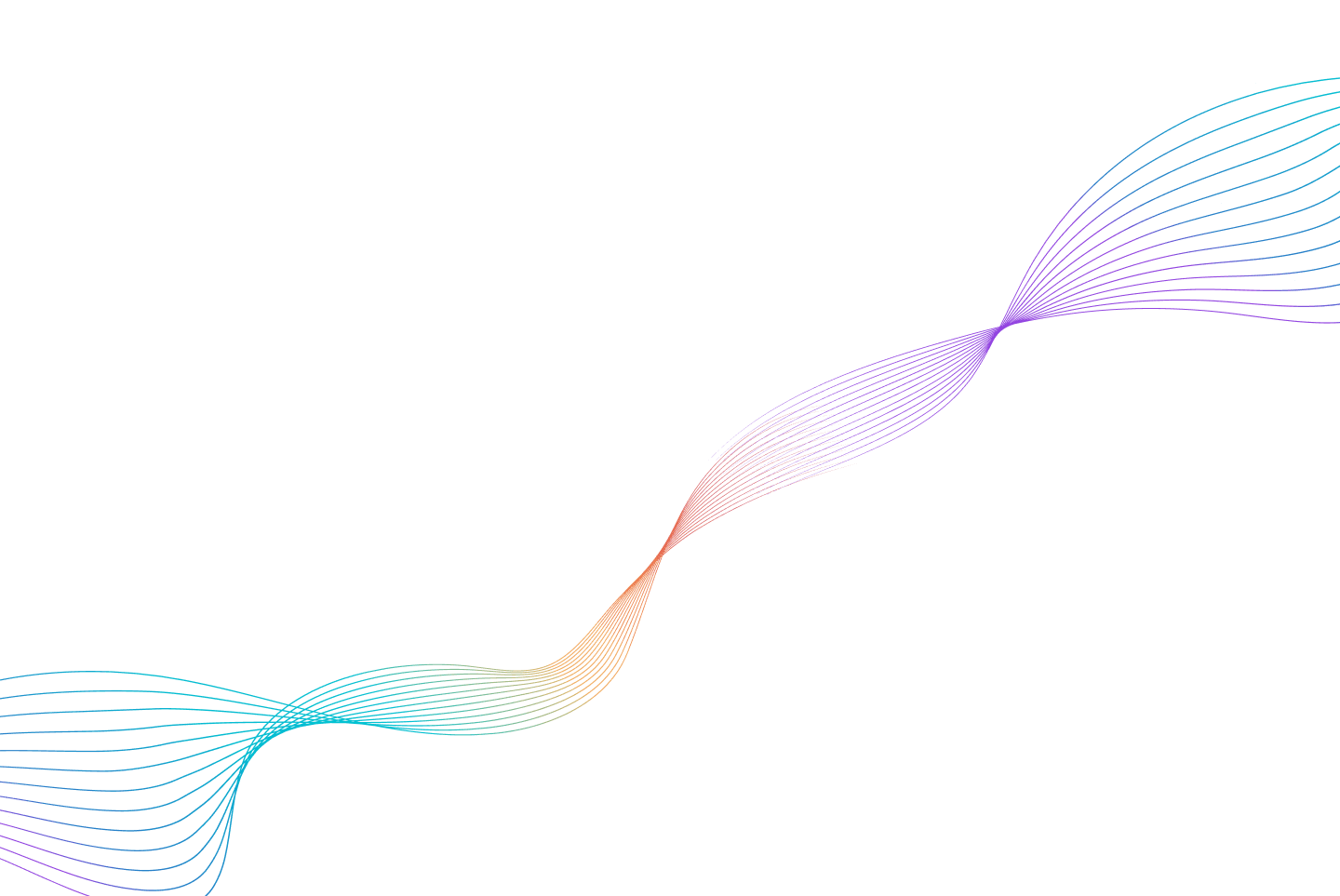