INSIGHTS

7 min read
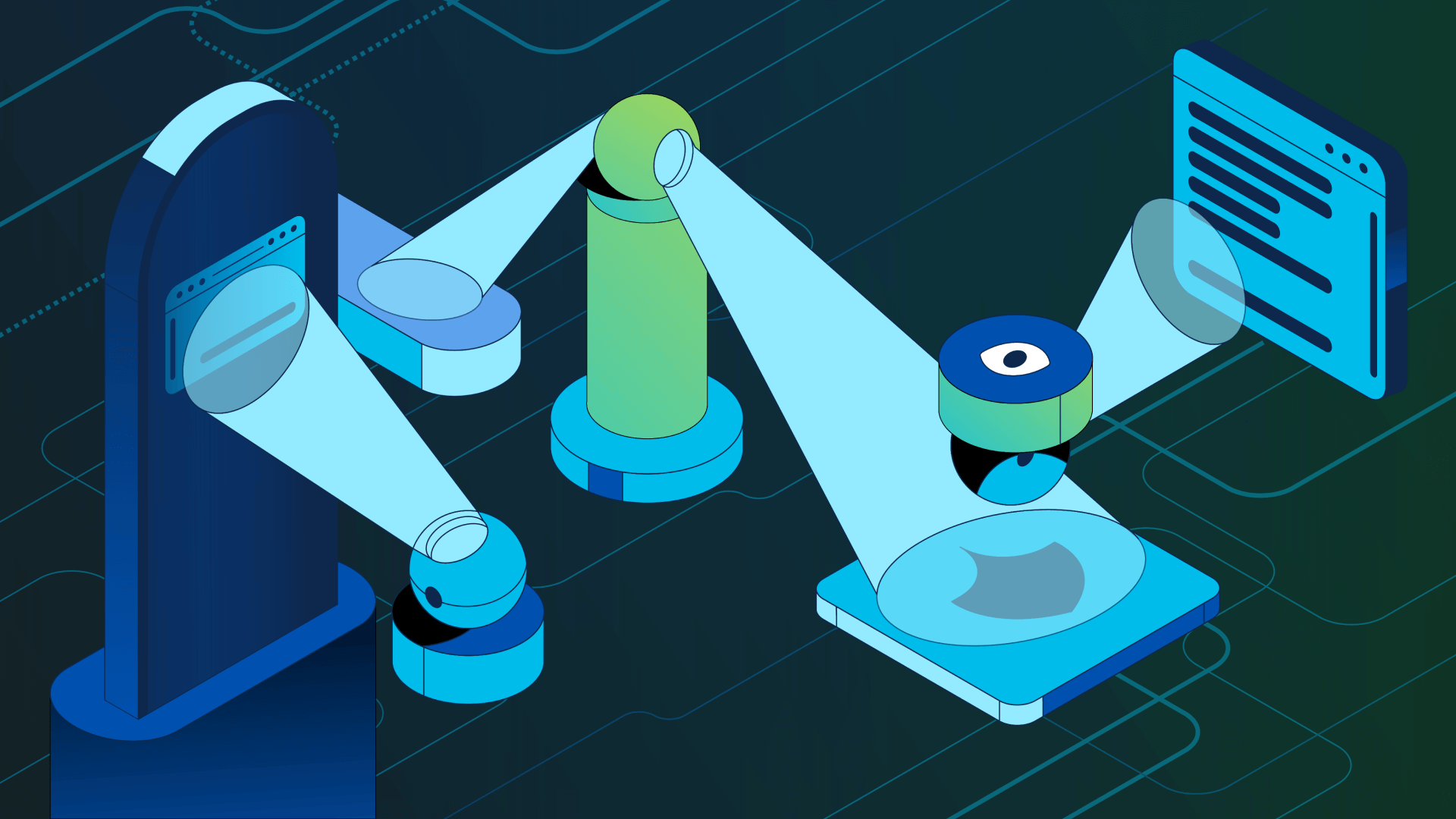
Published on 06/07/2024
Last updated on 06/18/2024
Responsible enterprise LLM challenges: Addressing compliance with privacy laws (Part 3)
Share
Establishing responsible practices related to large language models (LLMs) is crucial for enterprise success. Part of responsible LLM adoption includes using techniques to optimize output accuracy and reliability, as well as keeping model training data safe and secure. These strategies will allow you to get the most out of your enterprise LLM applications. However, they also help ensure compliance with laws and regulations meant to protect user data privacy, reinforcing stakeholder trust in your organization.
An overview of privacy and copyright regulations
While data is one of the most valuable assets available to any company, enterprises must now comply with regional legislation when handling third-party information.
According to Ivan Padilla Ojeda, a technical marketing engineer at Outshift by Cisco, the focus should be on data privacy legislation, “The most important laws and regulations are the ones related to the privacy of your customers.”
Leading global organizations committed to data protection and fostering stakeholder trust follow laws like the General Data Protection Regulation (GDPR) in the European Union (EU) and the California Consumer Privacy Act (CCPA), among others. Broadly speaking, these documents are intended to protect the rights of individuals whose information may be used for commercial purposes.
These principles also apply to enterprise LLMs. Trained with vast datasets from sources like social media, the web, and proprietary business data, LLMs often ingest data that contains sensitive information including names, addresses, and other personal content. Some LLMs may also save users’ prompts for training purposes. Organizations must consider any privacy laws that apply to this data used in pre-training or gathered during model deployment.
For example, imagine an LLM trained to take notes during surgery at a clinic in San Francisco. If the model generates notes including a patient’s personal information—like medical history and symptoms—this process must comply with any state or federal regulations protecting patient privacy, such as the Health Insurance Portability and Accountability Act (HIPAA), as well as the CCPA.
Your organization should take account of all the relevant laws and regulations when using LLMs, depending on where it operates and from whom it gathers data.
General Data Protection Regulation (GDPR)
The GDPR is considered the world’s strictest privacy and security law, outlining obligations for any enterprise that collects data belonging to or relating to EU citizens. Enterprises using LLMs must disclose what data is being used by the model as well as its purpose and method of use. Other considerations when staying GDPR-compliant include:
- Data transfers. Enterprises may be required to ensure that data originating from the EU stays there or is only transferred to countries with sufficient data protection rules in place.
- Data storage. The GDPR mandates that personal data isn’t stored for longer than necessary, and can be corrected or deleted on request.
- Data processing. Consent from data subjects may be required when using personal data for enterprise LLMs.
- Data security. Enterprises must implement sufficient security measures, under GDPR standards, to protect personal data used by LLMs.
EU AI Act
The EU AI Act was approved in February 2024 by the Council of the European Union, and ensures that artificial intelligence (AI) systems used in the EU are “safe, transparent, traceable, non-discriminatory, and environmentally friendly.” Under this Act, enterprises must acknowledge when content is generated by an LLM (as opposed to a human) and explain how the model was trained. Models should also respect copyright laws and use safeguards to ensure that outputs are lawful. In addition, organizations are obligated to report serious incidents to the European Commission when using general-purpose models like GPT-4.
California Consumer Privacy Act (CCPA)
The CCPA applies to for-profit enterprises doing business in the state of California. It gives consumers the right to know what personal data a business collects and how it’s used. As with the GDPR, enterprises must be able to access, delete, or correct personal information collected by the LLM (during either training or deployment) at a consumer’s request. They should also be transparent about what data the LLM collects and how it uses that data.
LLM architecture can make compliance challenging
Because of the way LLMs work, staying compliant with these regulations can get complicated. Large models are often considered “black boxes,” meaning that their internal functions lack transparency. It can be difficult to determine why a model may arrive at certain outputs, which makes it hard to communicate how a person’s information may be used.
There’s also the issue of honoring a person’s right to be forgotten included in regulations like the GDPR or CCPA, where organizations may be required to delete personal data. Even if content is erased from an LLM’s training data, the model’s initial training is already complete, leaving the potential for erased content to continue informing outputs. Although some developers have techniques to anonymize training data, achieving total anonymity is challenging.
Strategies to ensure compliance when using enterprise LLMs
Emerging AI and data privacy laws, combined with rapidly progressing LLM technology, make for a complex regulatory environment. Every organization will use third-party data in slightly different ways with large language models, so there’s no single road to compliance. However, some general strategies can help you stay ahead.
Understand relevant laws and partner terms
For many enterprises, the logical first step is to evaluate what data is used to train the LLM and where the owners of that data are located. In other words, determine which data privacy and AI laws and regulations apply to your organization and its LLM use cases.
It’s also important to carefully review the terms and conditions of your LLM technology partners—such as cloud, data, or model providers—to understand how they use data. For example, ChatGPT currently allows OpenAI to gather user inputs as training data, while the chatbot’s application programming interface (API) lets you opt out of this.
Draft a data processing agreement
Some enterprises may also benefit from creating a data processing agreement (DPA) that outlines roles and responsibilities between you and your providers. A DPA establishes policies around factors like data processing, storage, and transfers, which is important for compliance with laws like the GDPR. Educating employees, customers, and other stakeholders on your DPA terms can also foster transparency and keep LLM users aware of how their data is used and what their rights are.
Build compliance into your LLM systems
On the technical side, following LLM security best practices—like anonymizing training data and validating outputs for confidentiality—can help avoid privacy breaches that would violate regulations. Organizations can also develop techniques to ensure the LLM remains compliant depending on where it’s delivering outputs.
Padilla believes that the best approach is to develop a policy system that tells the LLM to exclude certain information before it is sent to the public LLM, on a case-by-case basis, when it would otherwise violate regulations.
“You need a policy control point that allows you to customize for particular situations and determine which types of information you’re allowed to disclose,” he says. “It’s not the same if you’re in the U.S., Asia, or Europe, so you need to customize those rules and apply them to different collectives.” For example, your organization may create policy rules so that your LLM is GDPR compliant if you have customers or users in the EU.
Integrating compliance with AI innovation is key to success
As enterprise LLMs become more common, more guidelines will likely emerge surrounding AI governance and data privacy. It’s important to use LLMs in accordance with laws that are relevant to your operating areas and educate your teams on rules and best practices. However, leading organizations will go above and beyond the minimum required to stay compliant, setting an ethical standard for responsible AI management and AI innovation as this technology transforms the way data is used.
Explore strategies for maximizing the potential of large language models in your enterprise. Learn how to ensure reliable, accurate, and unbiased outputs while navigating the challenges of AI compliance and data security.
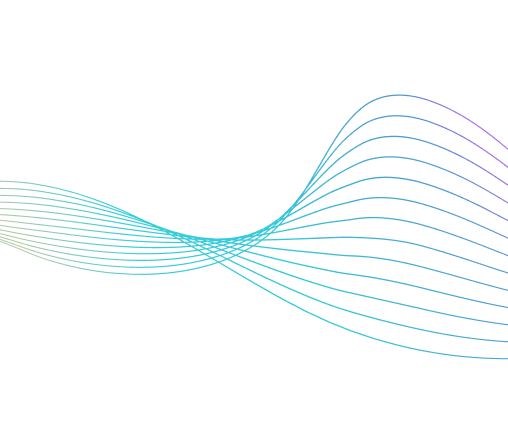
Get emerging insights on emerging technology straight to your inbox.
Unlocking Multi-Cloud Security: Panoptica's Graph-Based Approach
Discover why security teams rely on Panoptica's graph-based technology to navigate and prioritize risks across multi-cloud landscapes, enhancing accuracy and resilience in safeguarding diverse ecosystems.
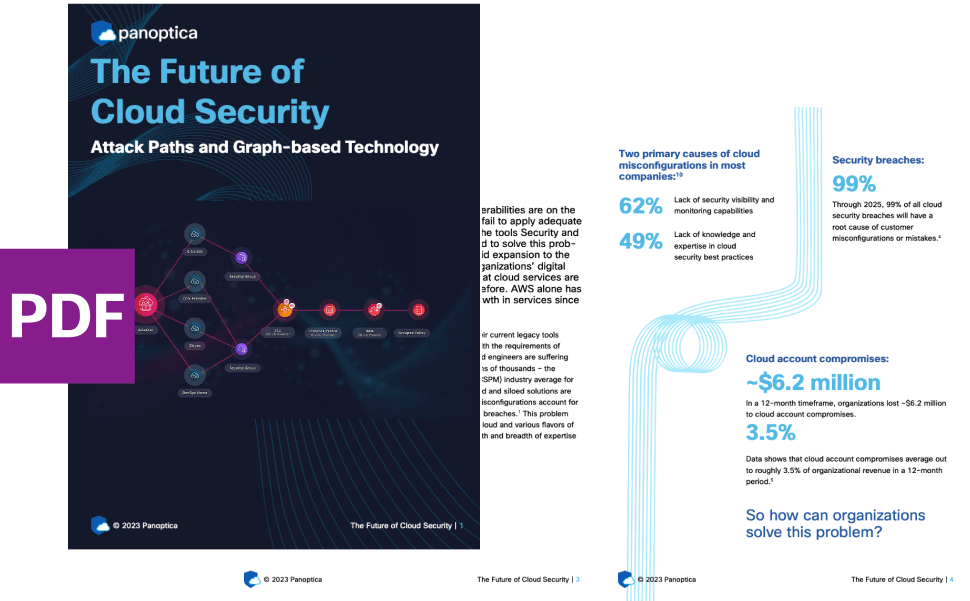
Related articles
The Shift keeps you at the forefront of cloud native modern applications, application security, generative AI, quantum computing, and other groundbreaking innovations that are shaping the future of technology.
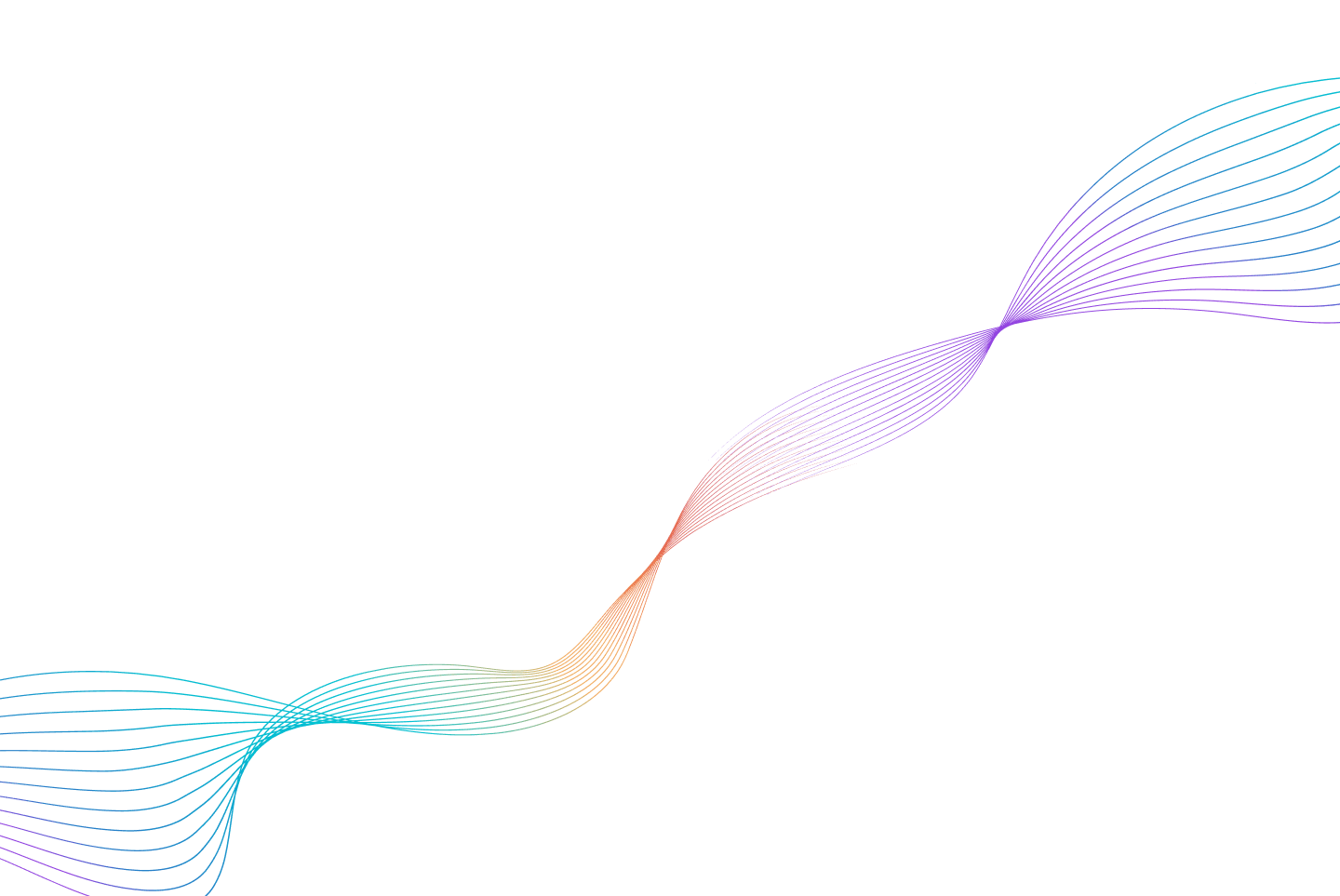